Executive Summary (tl;dr)
- Machine Learning brings the technical leverage needed to squeeze every drop of efficiency, but only if enough learning scale
- Narrowing the variability brings the majority of value - in this case tighter confidence interval for expected lifespan of "wear out period" assets
Introduction
The utilities industry is characterized by its asset-heavy nature. The problem with assets is as simple as time itself - they age. Infrastructure ages and operational demands evolve. Amidst this complexity, artificial intelligence solutions are being designed to keep pace with the needs of asset management practices. Utilities today demand advanced asset management strategies to help squeeze every possible efficiency from assets.

The "wear out period" in the Asset Management Lifecycle
The Harris Williams T&D Infrastructure report reveal a sobering reality - a significant portion of distribution assets within US utilities operate beyond their expected useful life. With approximately 30% of these assets nearing or surpassing their anticipated lifespan, utilities are currently involved in painful proactive asset management. This statistic underscores the criticality of implementing robust strategies to ensure the reliability and resilience of utility networks amidst aging infrastructure.
As assets enter the "wear-out period", typically beyond the 50-year mark, the frequency of failures escalates, posing substantial operational and financial risks to utilities. In this context, the importance of diligent asset management cannot be overstated. With each passing year, the exponential increase in failures underscores the need for utilities to adopt a cautious and proactive approach to asset management. By leveraging advanced technologies and data-driven insights, utilities can mitigate risks, optimize resource allocation, and safeguard service continuity. As we know, customers, both residential and business, care more about service continuity than they do anything else! Reliability is a Key Purchase Criteria (KPC) in the Utilities world.
Traditional asset management relies heavily on manual human monitoring, often overlooking complex fault patterns hidden beneath the surface. That's not to say that manual testing methods aren't important. Expensive assets, such as power plants, benefit greatly from routine manual testing. But what about the smaller assets? Is it best to let small assets, as a basket, follow a 3-strikes rule?
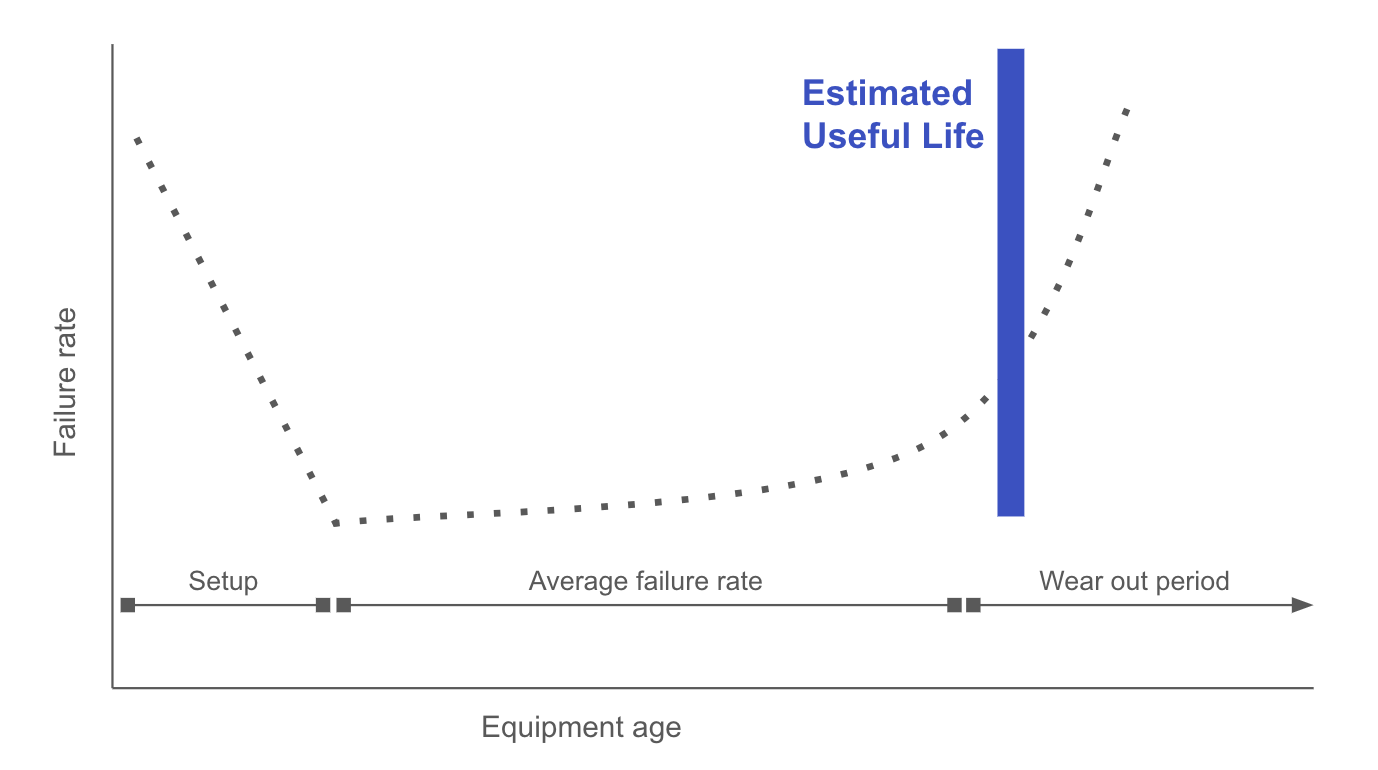
Machine learning recognizes hidden patterns
Enter machine learning – a game-changing solution to complement conventional monitoring methods. By harnessing the power of machine learning, utilities can enhance asset reliability, minimize downtime, and optimize maintenance schedules, thereby ensuring the longevity and efficiency of critical infrastructure.
Senpilot's AI-driven predictive analytics capabilities enable utilities to detect intricate fault patterns that evade human detection, facilitating early intervention and risk mitigation.
The goal of a concrete ML system, or any advanced model, should be to learn as much as possible. Think of the smartest asset manager you know - they're likely excellent at what they do because they have seen plenty of failures. Failures are output events. The post mortem research into the causes are the input events. Seeing more pairs of input and outputs can make a human better at predicting events. Similarly with models, more data will improve prediction accuracy. Back to the smartest asset manager - imagine cloning them 1000x and having them work at every Utility in the world. It's fair to say that the superstar asset manager will recognize patterns you've never seen.
Variability is the root of value
One of the inherent challenges faced by utilities is the variability in asset life expectancy across different operators. Just as personal vehicles exhibit varying lifespans based on maintenance and usage patterns, utility assets too display disparities in longevity. A strong model should be able to benchmark asset health given as much information as possible - the maintenance records, the manufacturers involved, the data of connected assets, etc. Through benchmarking, we can minimize the range of life expectancy, narrowing in the variability. It's easier to manage assets when asset life windows are better known! Minimizing variability enables better planning, the root of value.
By analyzing historical data, baseline industry performance metrics, and maintenance records, Senpilot empowers utilities to make informed decisions, maximize asset lifespan, and optimize capital expenditure.
Conclusion
As the utilities industry stands at a crossroads of technological innovation and operational optimization, we're building Senpilot to be a catalyst for change. By integrating artificial intelligence into asset management practices, utilities can unlock new levels of efficiency, resilience, and sustainability. Senpilot remains committed to driving positive change within the utilities sector, spearheading digital innovation, and empowering utilities to navigate the complexities of asset management with confidence and foresight.