Executive Summary (tl;dr)
- Predictive maintenance through the use of AI benefits operations in 3 key aspects:
a) Reduced Downtime & Increased Reliability (up to 60%)
b) Cost Savings (15% on average / asset)
c) Extended Equipment Lifespan (up to 50%) - Best practices to implement predictive maintenance include:
a) Create a robust data collection process and use software to help with collection
b) Leverage machine learning to interpret data and constantly update the model
c) Foster a culture of continuous improvement and collaboration across teams
What is predictive maintenance?
No matter how well analysts predict last night's basketball game, anytime human judgment is involved the prediction is subject to personal biases and errors. In relation to asset maintenance, these personal biases along with the need to factor in millions of data points regarding asset health are key in order for a Utility (or any capital intensive industry) to ensure that they are operating at peak efficiency.
Predictive maintenance is a proactive approach to maintaining equipment and infrastructure by using data to predict the schedule of when maintenance should be completed to perform at peak efficiency. This is achieved through monitoring the condition of equipment through sensors and real-time data collection as well as referencing historical evidence of similar systems. Additionally, millions of external factors such as asset maintenance history, installation conditions, connected assets health, etc. are utilized in machine learning models to predict ideal maintenance schedules.
A strong predictive model allows for early detection of potential issues before they lead to failures. By predicting maintenance needs, organizations can reduce downtime and increase reliability of assets, save on costs and extend the lifetime of their equipment.
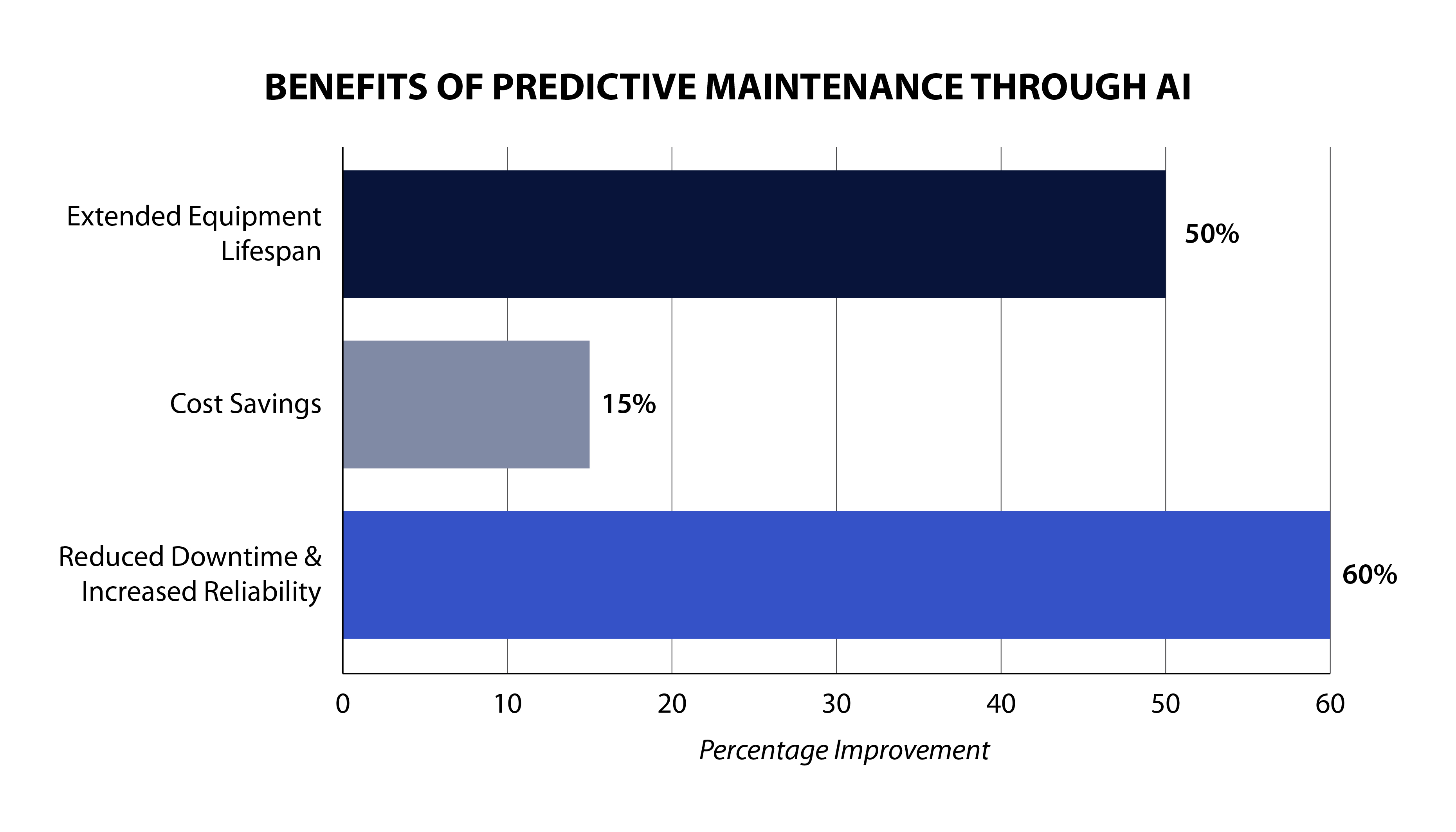
A) Reduced Downtime & Increased Reliability
Predictive maintenance helps identify potential failures before they occur. By analyzing data from equipment sensors and historical performance, Utilities can predict when a component is likely to fail and perform maintenance just in time to prevent it. These models get stronger over time with more data points and this leads to fewer and fewer unexpected outages and increased reliability of the utility services.
B) Cost Savings
By preventing unexpected breakdowns and reducing the need for emergency repairs, predictive maintenance can lead to substantial cost savings. It allows for the most efficient use of maintenance resources and minimizes the expense of major repairs by addressing issues early. Moreover, it helps in optimizing the inventory of spare parts, reducing holding costs and labour costs to fix issues.
C) Extended Equipment Lifespan
Just like scheduled maintenance on a car, regular and timely maintenance based on predictive analytics can extend the life of utility equipment by over up to 50%. By ensuring that components are serviced or replaced at the optimal time, utilities can avoid the excessive wear and tear that comes from operating equipment until failure. This not only enhances the performance and reliability of the equipment but also delays the need for costly replacements.
Best practices for Predictive Asset Maintenance
How do you ensure you apply the best practices for predictive maintenance to get the best out of all your assets? There are a few key strategies to ensure effectiveness.
Firstly, it's crucial to implement a robust data collection system that utilizes sensors and IoT devices to continuously monitor equipment conditions. This data should be analyzed using advanced analytics and machine learning algorithms to accurately predict potential failures. Regularly updating, maintaining and feeding the predictive models is essential to adapt to changing conditions and improve accuracy. Additionally, integrating predictive maintenance into a comprehensive asset management plan helps prioritize maintenance tasks and allocate resources efficiently. Finally, fostering a culture of continuous improvement and collaboration across departments can drive innovation and optimize the overall maintenance strategy.
Conclusion
Leveraging a predictive model for asset maintenance is vital in ensuring you are running assets in the most effective and efficient manner possible. Using a strong AI or machine learning model with predictive maintenance will lead to more accurate predictions on schedules as these models improve on themselves. These improvements lead to increased reliability with a reduction in downtime, cost savings and can lead to a 50% extension in the life of equipment.
The more you use a predictive model, the better it gets. It's a positive feedback loop that every Utility must leverage to stay abreast in a data intensive world.